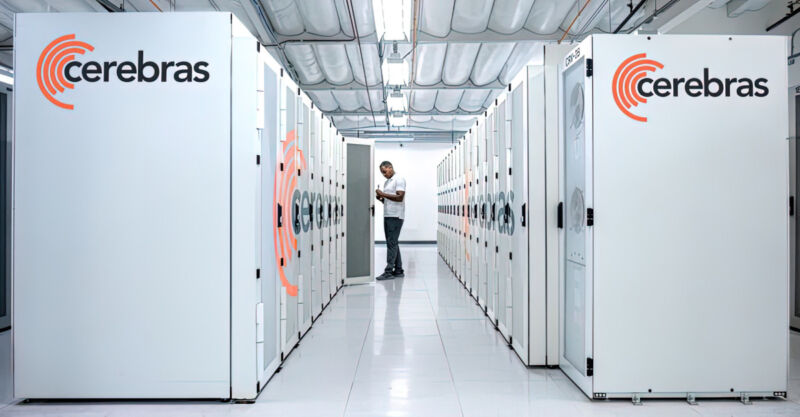
On Monday, Cerebras Systems unveiled its 13.5 million core Andromeda AI supercomputer for deep learning, reports Reuters. According Cerebras, Andromeda delivers over one 1 exaflop (1 quintillion operations per second) of AI computational power at 16-bit half precision.
The Andromeda is itself a cluster of 16 Cerebras C-2 computers linked together. Each CS-2 contains one Wafer Scale Engine chip (often called “WSE-2”), which is currently the largest silicon chip ever made, at about 8.5-inches square and packed with 2.6 trillion transistors organized into 850,000 cores.
Cerebras built Andromeda at a data center in Santa Clara, California, for $35 million. It’s tuned for applications like large language models and has already been in use for academic and commercial work. “Andromeda delivers near-perfect scaling via simple data parallelism across GPT-class large language models, including GPT-3, GPT-J and GPT-NeoX,” writes Cerebras in a press release.
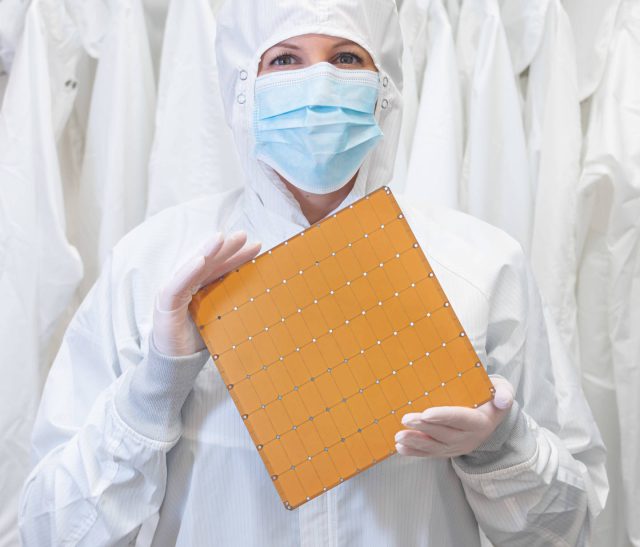
The phrase “Near-perfect scaling” means that as Cerebras adds more CS-2 computer units to Andromeda, training time on neural networks is reduced in “near perfect proportion,” according to Cerebras. Typically, to scale up a deep-learning model by adding more compute power using GPU-based systems, one might see diminishing returns as hardware costs rise. Further, Cerebras claims that its supercomputer can perform tasks that GPU-based systems cannot:
GPU impossible work was demonstrated by one of Andromeda’s first users, who achieved near perfect scaling on GPT-J at 2.5 billion and 25 billion parameters with long sequence lengths—MSL of 10,240. The users attempted to do the same work on Polaris, a 2,000 Nvidia A100 cluster, and the GPUs were unable to do the work because of GPU memory and memory bandwidth limitations.”
Whether those claims hold up to external scrutiny is yet to be seen, but in an era where companies often train deep-learning models on increasingly large clusters of Nvidia GPUs, Cerebras appears to be offering an alternative approach.
How does Andromeda stack up against other supercomputers? Currently, the world’s fastest, Frontier, resides at Oak Ridge National Labs and can perform at 1.103 exaflops at 64-bit double precision. That computer cost $600 million to build.
Access to Andromeda is available now for use by multiple users remotely. It’s already being utilized by commercial writing assistant JasperAI and Argonne National Laboratory, and the University of Cambridge for research.